Raiffeisenbank ran a 2-month pilot with 14 active users.
Users received guidance on weekly basis and workshops.
We validated business demand as well as accuracy.
Lakmoos provided 25 research projects.
Deep dive
Overview
Lakmoos at Raiffeisenbank
Today was a big day for us! 🎉
We kicked off our pilot with Raiffeisenbank Česká republika.
Company overview
Raiffeisenbank (RB) is a prominent financial institution operating on the Czech market. Known for its comprehensive range of banking and financial services, Raiffeisenbank serves millions of customers, including individuals, small and medium enterprises (SMEs), and large corporations. The bank prides itself on its innovative approach to banking, integrating advanced technologies to enhance customer experience and operational efficiency.
Climat
Motto of RB is “A bank inspired by clients”. RB has developed a culture of interacting with clients and putting them first. In 2024, the banks’ quest is to explore how to leverage AI to take customer-centricity an extra mile.
Time
Q2 2024
Research projects done
25 (1 Quantitative survey and 24 Qualitative interviews)
Timeline
Main goal
Validate the quality of answers on custom use cases
Overcome skeptical voices with testing and trying
Create business demand
Pilot topic
Loyalty programs
Secondary topics: mortgage, cards, parent-child banking habits and attitudes
People involved
Key stakeholders: 3
Active users: 14
Met us at least once: 11
Departments: Innovation, Strategy, CX
Roles: product owners, research managers, strategy managers
Who participated
Heroes of the project
The biggest hero of all is Andrea who gave us the opportunity and endorsement to execute our wild plan. Here is to you, we would be nothing without our champions!
1
Recruitment
It is hell to recruit people for custom interviews and the return rates on surveys are terrible, the last one was at 0.38%.
With Lakmoos, we can reach anyone anytime.
2
Speed
It takes ages to go from need to result. Although data collection takes 2 weeks, the process around it lasts another 5 months.
With Lakmoos, we can fire questions anytime and get instant results.
3
Testing the small stuff
Today, there is only budget for research for big projects and big decisions. That often keeps key stakeholders in the dark and we have no option but to make an educated guess which is still a guess.
With Lakmoos, we have unlimited access to the clients’ opinions and can test everything.
Překvapilo mě, že to nebylo robotický, ale že jsem měla pocit, jako by to byla fakt diskuse s opravdovým člověkem. Působilo to tak na mě.
Zuzana, strategy
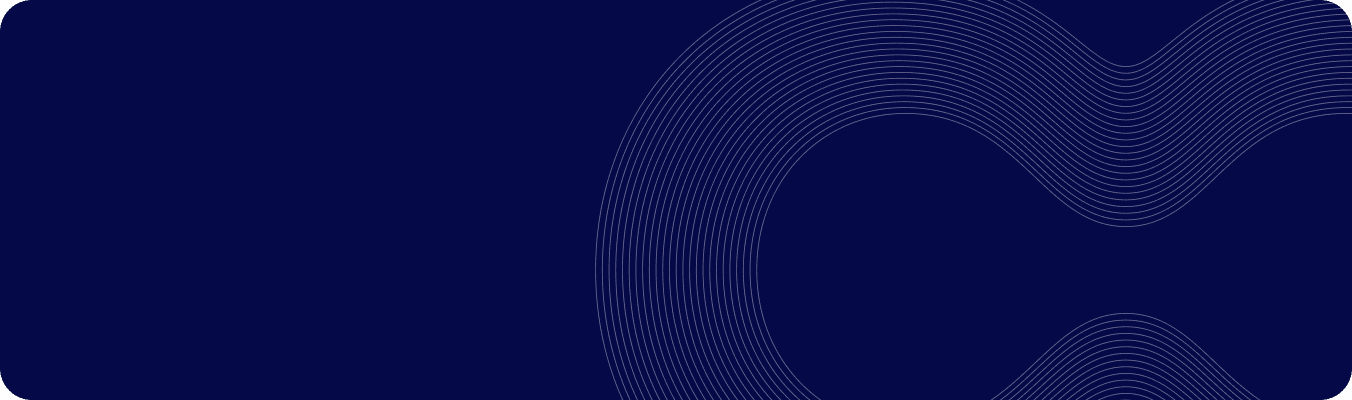
What we delivered
Results
224 questions asked
After consulting with RB team, we created three strategic personas that represent wider groups of 41k-78k people. Besides demographic info, we filtered these people by lifestyle and banking behaviour.
Most active - 95 questions
Least active - 2 questions
Mean - 17.42 questions per each active user
The best questions in Interviews
Do you need to test mobile application before you decide on bank change?
Are there any benefits or services you would like to see included in the loyalty program?
Do you save up and how?
How do you feel about gamification elements (e.g., challenges, tiers, badges) in a loyalty program?
What bank accounts do you have?
In Qualitative interviews, users could ask questions and get a Group Fit indices (How many people from the group agree with this answer?) and Sentiment (Is this an important topic? What emotions does it stir up?)
Survey example: Loyalty programs
32 Likert scales (4-point)
2 Open-ended questions
1 Multi-choice question
Brief example
Raiffeisenbank is preparing a loyalty program for its clients. The program is based on completing tasks and challenges, for which the client can then receive a reward.
The research will help us innovate and revise our current loyalty program and design challenges that will be attractive to the majority of our client base.
I want to ask the clients of Raiffeisenbank.
In Quick Questions, users could ask questions and get a Group Fit indices (How many people from the group agree with this answer?) and Sentiment (Is this an important topic? What emotions does it stir up?)
User engagement was encouraged in RB Teams. We monitored bad questions and suggested correct format, wording or topic.
Validation showed that the model gets better with more data. I can imagine to upload 10 questions and ask about the 11th. I would still be hesitant to do a full AI research without adding any more data and present it at the Board, though. We will continue to test the model as it develops and integrates more data.
Robert
First reality check in Qualitative interviews
Why do we test in more steps? We need to validate two things: 1. our data baseline is not complete garbage and 2. we can plug in your data to make the model up to 1000x more accurate.
RB researchers tested obvious inconsistencies with Qualitative interviews, where individual answers seemed plausible.
So we digged deeper and simulated the whole survey in Quantitative survey.
Baseline model vs. real survey
Baseline model accuracy: 70.20% weighted order match on likert scale questions
Baseline model = model did not see any data from RB.
RB chose a survey on their clients from May 2024 (i.e. two months prior to our pilot) with > 900 responses on Likert scales, multi-choice questions and open-ended questions.
We first compared the order of suggested benefits measure by the Likert scales.
Then, Lakmoos corrected for anomalies in RB sample, e.g. above-average age.
We also provided system data without all the shiny colours.
AI respondents combine both aspects of research: quantitative (percentages and distributions) and qualitative (reasons and quotes).
Enriched model vs. real survey
Model enriched with only subset of data: 88.94% weighted order match on likert scale questions.
Enriched model = model saw part of the data, incl. distribution and answers to some questions.
Lakmoos corrected for anomalies in RB sample, e.g. above-average age.
RB shared ranking of 15 out of 30 benefits and GDPR-compliant demographic info of the group.
Lakmoos linked the new information with the model.
How accurate will the answers be?
Can we start the web app on your work PCs?
Will anyone use it?
-
Data integration and compliance
-
Full research with no prior data
Before a RB data layer is built, the model excels at reliable Qualitative interviews, but the range for full research (Quantitative survey) is restricted to shared pieces of data.
More testing of full research will be done during the ramp-up phase to determine to what extent and in what topics Quantitative survey could run full research.